Yan Xing, MD, MS1, Asha S. Kapadia, PhD
2, Janice N. Cormier, MD, MPH
1, John M. Swint, PhD
2, and Claudia Pedroza, PhD
2. (1) The University of Texas M.D. Anderson Cancer Center, Houston, TX, (2) The University of Texas School of Public Health, Houston, TX
Purpose: To provide more flexible approaches for meta-analysis of diagnostic tests by modeling the actual observed outcomes using two Bayesian generalized linear models (GLM). Methods: The data from a published meta-analysis, which compared the results of three imaging techniques, lymphangiography (LAG), computed tomography (CT), and magnetic resonance imaging (MRI), for the diagnosis of lymph node metastasis in cervical cancer patients, was used to illustrate two Bayesian approaches. In the bivariate binomial model (BBM), pairs of sensitivity and specificity values were jointly analyzed while incorporating the correlation between these two outcome variables. Noninformative independent uniform priors were assigned to the covariance matrix. In the multinomial model (MNM), test results were modeled as multinomial random variables. Both models included the imaging techniques as covariates to compare performance between three different diagnostic tests. Statistical computations were conducted in WinBUGS 1.4.2. Results: The point estimates from the two models for CT and MRI were similar, while those for LAG were lower in the MNM than in the BBM (Table). The MNM yielded a narrower 95% credible interval (CI) than BBM. A sensitivity analysis showed that the BBM was robust with regard to the prior distribution of the covariance matrix. Both point estimates and 95% CI of Bayesian BBM are similar to the result obtained by its frequentist counterpart. Conclusions: Bayesian GLM provide a realistic, flexible framework for incorporating uncertainty in the meta-analyses of diagnostic tests. In addition to facilitating direct comparison between different tests, these models capture the variability in both sensitivity and specificity simultaneously as well as the intercorrelation between the two. Both the BBM and MNM give similar point estimates, however the MNM gives tighter credible intervals and is easier to implement in WinBUGS.
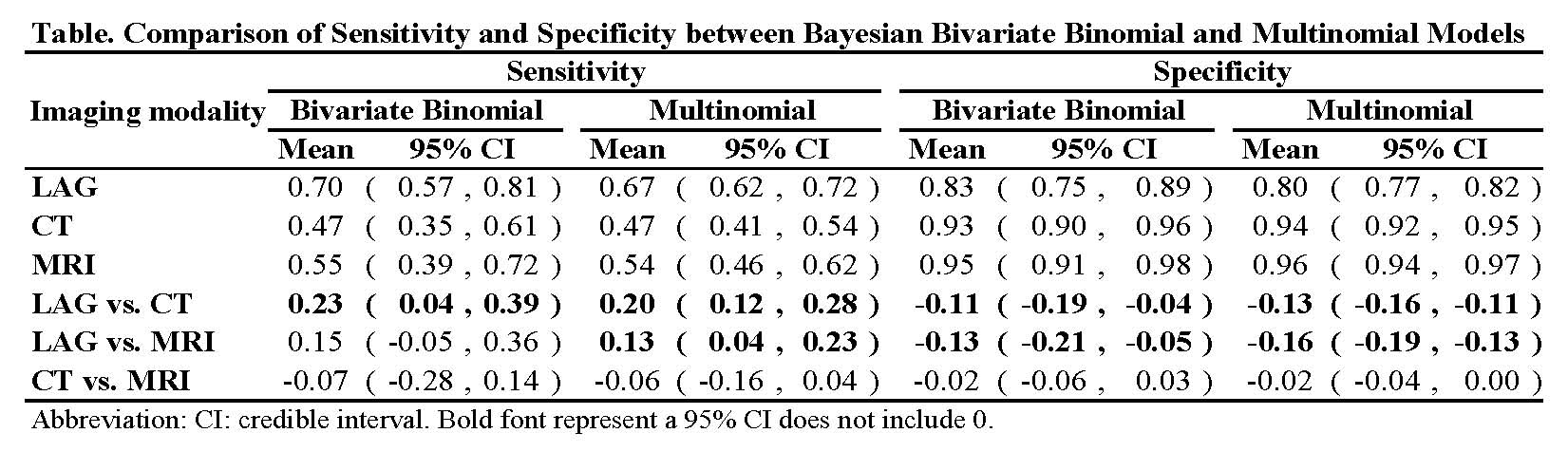